The Uncomfortable Truth: Why Your AI Strategy is Only as Good as Your Data
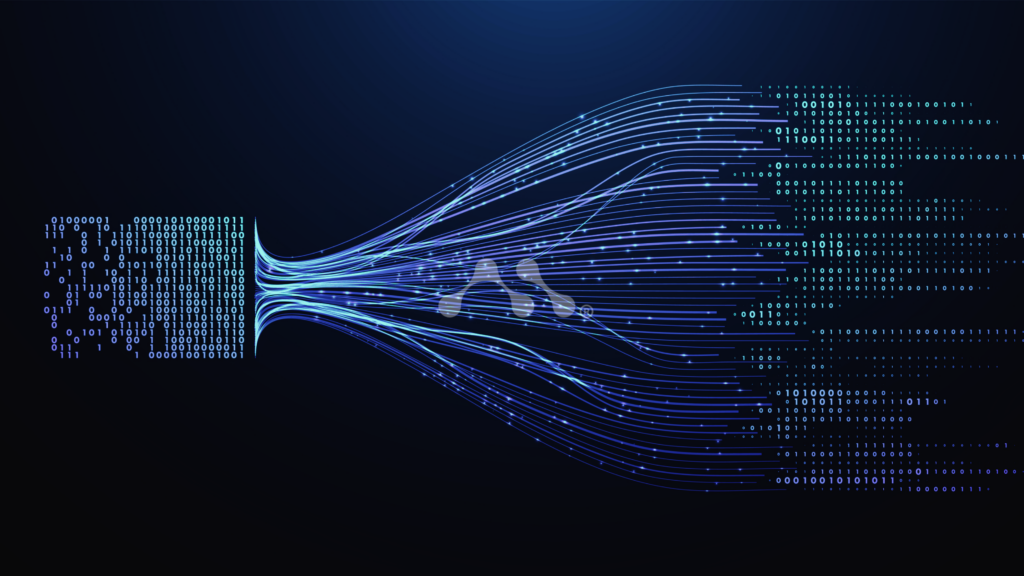
Let’s Have a Real Conversation
Remember the line “Help me help you” from Jerry Maguire?
It’s time we apply that same principle to the current AI gold rush, especially around Generative AI. While boardrooms and Slack channels buzz about AI strategies, there’s a blunt reality few want to confront: without a world-class data strategy, your AI ambitions are just castles in the sand.
The Hype Around Generative AI
Since ChatGPT’s breakout in late 2022, it’s been a Wild West moment for AI. Gartner reports a 1500% increase in enterprise AI project launches between 2023 and 2025.
Sequoia Capital calls it “the fastest adoption of a consumer technology in history.”
It seems every business today has an AI pilot—or ten—in production. But the uncomfortable question remains:
Are we building sustainable value, or just chasing headlines?
Data: The Unsung Hero—or the Achilles’ Heel
AI without data is like a Ferrari without fuel.
That sounds obvious—but most organizations underestimate the difference between having data and having the right data.
Accenture recently found that only 27% of companies feel they have “highly reliable, trustworthy, and actionable” data for AI initiatives.
Yet without clean, enriched, contextually relevant datasets, the most sophisticated models will still produce noise—or worse, confident inaccuracies.
In other words: bad data doesn’t just slow you down—it actively misleads you.
The Dirty Secret: Data Integration and Governance
Everyone wants to fine-tune LLMs or build custom copilots.
But few want to roll up their sleeves and fix the real problem: fragmented, poorly governed, legacy data infrastructures.
A McKinsey report warns that 60-80% of AI project time is spent just on cleaning, preparing, and integrating data.
Yet too often, executives treat data governance as a boring afterthought—only to watch promising AI pilots fail because their foundations were rotten.
In the AI race, quantity of data matters—but quality of data determines who crosses the finish line.
The NLP Illusion: Are We Fooling Ourselves?
Natural Language Processing (NLP) powers much of the magic behind today’s GenAI applications.
But here’s the trap: having pipelined data ready for LLMs isn’t the same as having useful, accurate, bias-mitigated data.
If your training corpus is riddled with outdated, incomplete, or context-poor data, you’re not building intelligence—you’re building delusion.
As MIT’s Professor Regina Barzilay puts it:
“AI is only as good as the examples it sees. And most corporate datasets were never designed for intelligence—they were designed for compliance.”
Generative AI: The Double-Edged Sword for Data Governance
Generative AI isn’t just consuming data; it’s beginning to reshape it—and that’s a risky feedback loop.
- Intelligent Data Quality: AI can spot missing fields—but it often lacks human nuance. Garbage in, garbage out still applies.
- Synthetic Data Generation: Yes, synthetic datasets can expand models—but if not carefully validated, we risk creating echo chambers of bad assumptions.
- AI-Driven Governance: Automating data policies sounds efficient—but blind reliance can codify existing biases faster than ever.
- Self-Healing Systems: Letting AI “fix” its own data errors is elegant—but if a model misjudges an error, it compounds mistakes at machine speed.
In short: AI doesn’t absolve us from human judgment—it demands better judgment than ever before.
Conclusion: Time to Face the Music
The marriage between Data Strategy and AI Strategy is not optional—it’s existential.
A brilliant AI model running on compromised data isn’t an innovation. It’s an expensive illusion.
As we move deeper into the age of autonomous systems, our competitive advantage won’t just be model architecture or LLM fine-tuning. It will be data stewardship.
So the question is no longer “What’s your AI strategy?”
It’s:
“Is your data ready to meet your AI ambitions?”
Because at the end of the day:
👉 Your AI will never be better than your data.
And that’s the uncomfortable truth.
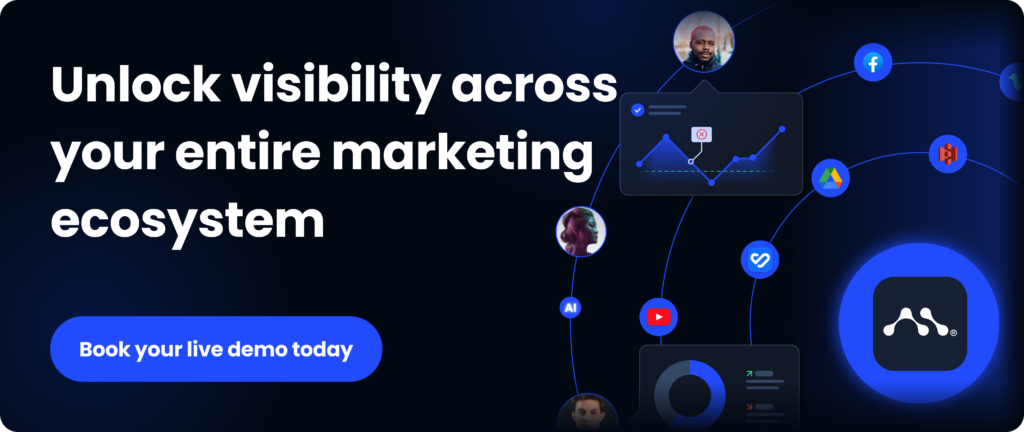